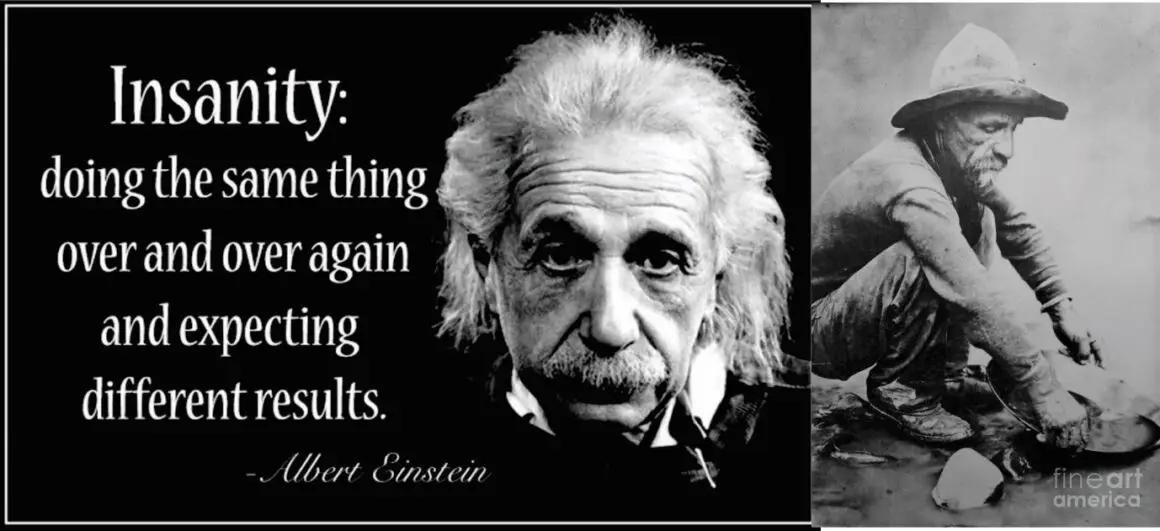
Insanity and Relevant Range: Implications for ML and AI
Insanity and Relevant Range: Implications for ML and AI
Einstein once said the definition of insanity is doing the same thing over and over again, expecting different results. On the surface, this makes sense. But perhaps it is like the difference between Newtonian/classical and quantum physics. Classical physics is a subset of quantum physics over a relevant range. The same can be said of planar and spherical geometry. Einstein’s quote needs to be amended to insanity is doing the same thing over and over again, expecting different results under the same conditions.
But what if conditions change?
Let us consider two examples. The first is relatively simple, and the second seems simple, but is actually complex.
The first is my son’s dog, Chance. When he visits, he expects to run several miles a day with me. From the moment he gets up, he hunts me down and pesters me until I take him for a run. He does the same thing over and over and gets the results he wants. But when we run, he sees a lot of squirrels and tries to chase them. Every time he takes up the chase of the squirrel and hits the end of his leash, and stops with a jerk. He does the same thing over and over again, expecting different results. But what if the leash slips out of my hand? The immediate conditions change, and he can chase the squirrel.
Now consider the gold panner. He pans over and over and gets some gold. But that only works on a stream or river. The water flow brings the gold from elsewhere. But if he pans over and over on a pond, he’ll probably never find gold. He meets Einstein’s criteria. The stream is fine; the pond is bad. But what if something happens to the stream or the source of the gold? Conditions change and the gold stops. He continues to pan for gold based on his history of success. But the condition change is outside of his sensory input and/or frame of reference. The relevant range changes and his experience may count for little or nothing. He may not even be aware of the conditions change because they are outside of his sensory range.
These two examples are simple compared to most operational and strategic issues, but they help to illustrate three key points that will affect an organization’s success with AI/ML and to avoid insane decisions.
- The organization must have clearly defined goals and objectives that guide actions. This presents a second facet of insanity Einstein did not consider: repeatedly conducting actions that are not aligned to goals and objectives.
- The organization must have a robust sensory network to gather data, organize it and turn it into actionable information based on the goals and objectives.
- The organization must have a well-developed assessment system to ensure that its actions are not insane and to discover the events and issues that will affect operations and strategy. This must include a set of well-defined standards and the collection capabilities to gather the information to assess them.
The overall system must be sensitive to relevant ranges and alert decision-makers when the data moves outside of the relevant range. When it does, data scientists need to assess the impact of the change and determine when to retrain the AI/ML. If the system does not adjust, it moves into a new level of insanity, which I’ll call robot insanity. This is relying on a system that can lead to poor and sometimes dangerous decisions.
I suspect that this third level of insanity may have led to the democratic route in the 2024 elections.
The question then, is, can we restore sanity to our systems? This will take a renewed emphasis on cognitive capabilities and critical thinking. Our education and media need a complete overhaul.